Decision Vision Episode 166: Should I Use Artificial Intelligence in my Business? – An Interview with Charles Wardell, Digital Cortex, Inc.
Exploring the evolution of artificial intelligence (AI) in general and specifically for business use, Charles Wardell, CEO of Digital Cortex, and host Mike Blake discussed how to define AI, machine learning, and its applications both commercial and otherwise. They also covered its impact on the pandemic, the social implications of AI, the need for a commitment to trustworthy data, and much more.
Decision Vision is presented by Brady Ware & Company and produced by the North Fulton studio of Business RadioX®.
Digital Cortex, Inc.
As technology rapidly evolves, so does the need for faster, more efficient data processing methods.
The Central Processing Unit (CPU) has been the workhorse behind digital endeavors, but today’s computation and data volumes challenge even the fastest CPUs. The modern world is increasingly becoming one where data reigns supreme. Data processing has evolved from serial computation and sequential storage to parallel computing with large pipelines and vast amounts of memory.
Today, there are options for accelerating computation, graphics processing units, FPGAs, ASICs, and DPUs designed specifically for data processing. Digital Cortex aims to converge these advanced technologies into a single unified platform, creating an ecosystem that simplifies the hyperscaling of complex data processing.
The Digital Cortex platform is a data appliance with built-in acceleration. It handles the undifferentiated heavy lifting so that you can focus on logic, analysis, and results. Our company is designed to bring the power of the Cloud to those use cases that cannot tolerate outage, latency, or uncapped expense.
Charles Wardell, CEO, Digital Cortex, Inc.
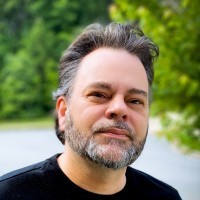
Charles Wardell, CTO, Tech Visionary, Maker of Things That Work Fast with decades of experience working with leading MPP databases to implement world-class BI platforms, and then designing and developing one of the world’s most powerful cloud and edge-based analytics engines using MPP (massively parallel processing), grid computing, ML (machine learning), and AI (artificial intelligence), Charlie routinely tackles some of the world’s messiest and most intractable problems. The fact is, Charlie is one of the best big data platform architects in the world.
His superpower is weaving hardware, software, and database technologies into cutting-edge, high-performance solutions that provide insights at the scale and speed modern businesses require. The breadth and depth of Charlie’s experience also enable him to see around the corners well in advance, and the combination of his and David’s vision targeted on the biggest and most valuable solutions is what makes this duo such an amazing team.
If intellectual curiosity was a degree, Charlie would have a PhD. His curriculum has been intensive, and it continues today, his library is extensive, not in one discipline, but several: hardware, software, and database technology. But beyond reading, Charlie’s best work comes out of his lab, whether it’s a customized FPGA, the fastest database in the world (measured by inserts), or it’s a new application that integrates symbolic and connectedness AI, there is nothing he can’t do. However, his best problem-solving characteristics are that he is a natural systems thinker and he never brings bias to a problem, every problem gets his full attention, so he can always focus on identifying the best tool for the job, not necessarily the tool he knows. This is what makes him one of the best architects on the planet, maybe the solar system.
Mike Blake, Brady Ware & Company
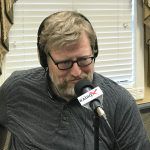
Michael Blake is the host of the Decision Vision podcast series and a Director of Brady Ware & Company. Mike specializes in the valuation of intellectual property-driven firms, such as software firms, aerospace firms, and professional services firms, most frequently in the capacity as a transaction advisor, helping clients obtain great outcomes from complex transaction opportunities. He is also a specialist in the appraisal of intellectual properties as stand-alone assets, such as software, trade secrets, and patents.
Mike has been a full-time business appraiser for 13 years with public accounting firms, boutique business appraisal firms, and an owner of his own firm. Prior to that, he spent 8 years in venture capital and investment banking, including transactions in the U.S., Israel, Russia, Ukraine, and Belarus.
LinkedIn | Facebook | Twitter | Instagram
Brady Ware & Company
Brady Ware & Company is a regional full-service accounting and advisory firm which helps businesses and entrepreneurs make visions a reality. Brady Ware services clients nationally from its offices in Alpharetta, GA; Columbus and Dayton, OH; and Richmond, IN. The firm is growth-minded, committed to the regions in which they operate, and most importantly, they make significant investments in their people and service offerings to meet the changing financial needs of those they are privileged to serve. The firm is dedicated to providing results that make a difference for its clients.
Decision Vision Podcast Series
Decision Vision is a podcast covering topics and issues facing small business owners and connecting them with solutions from leading experts. This series is presented by Brady Ware & Company. If you are a decision-maker for a small business, we’d love to hear from you. Contact us at decisionvision@bradyware.com and make sure to listen to every Thursday to the Decision Vision podcast.
Past episodes of Decision Vision can be found at decisionvisionpodcast.com. Decision Vision is produced by John Ray and the North Fulton studio of Business RadioX®.
Connect with Brady Ware & Company:
Website | LinkedIn | Facebook | Twitter | Instagram
TRANSCRIPT
Intro: [00:00:02] Welcome to Decision Vision, a podcast series focusing on critical business decisions. Brought to you by Brady Ware & Company. Brady Ware is a regional, full-service accounting and advisory firm that helps businesses and entrepreneurs make visions a reality.
Mike Blake: [00:00:22] Welcome to Decision Vision, a podcast giving you, the listener, clear vision to make great decisions. In each episode, we discuss the process of decision-making on a different topic from the business owners’ or executives’ perspective. We aren’t necessarily telling you what to do, but we can put you in a position to make an informed decision on your own and understand when you might need help along the way.
Mike Blake: [00:00:44] My name is Mike Blake, and I’m your host for today’s program. I’m a director at Brady Ware & Company, a full-service accounting firm based in Dayton, Ohio, with offices in Dayton; Columbus, Ohio; Richmond, Indiana; and Alpharetta, Georgia. I am managing partner of the Strategic Valuation and Advisory Services Practice, which brings clarity to the most important strategic decisions that business owners and executives face by presenting them with factual evidence for such decisions. Brady Ware is sponsoring this podcast.
Mike Blake: [00:01:12] If you would like to engage with me on social media with my Chart of the Day and other content, I am on LinkedIn as myself and @unblakeable on Facebook, Twitter, Clubhouse, and Instagram. I also recently launched a new LinkedIn group called Unblakeable’s Group That Doesn’t Suck. So, please join that as well if you would like to engage.
Mike Blake: [00:01:31] Today’s topic is, should I use artificial intelligence in my business? According to PEGA, 77% of people already use a device or service that is AI powered. Eighty-five percent of customer relationships with business enterprises will be managed without human involvement, according to Gartner. And, according to Forbes, the number of AI startups since 2000 has increased four times.
Mike Blake: [00:01:57] And, you know, I’m actually a little surprised we haven’t gotten to this topic until now. It’s such an important topic. And, AI and the things that go with it or talk about it today are so pervasive that to be candid, spoiler alert, I think we’re going to come away from this conversation not so much debating how one, whether one should incorporate AI into your business, but what is the best way to do it or what’s the feasible way to do it because, you know, it’s in everything.
Mike Blake: [00:02:30] So, if you’ve been sort of living with a fear or a notion of robots sort of taking over or taking over things in our society, I got bad news for you. It’s already been happening for about 15 years or so, if not longer. But, knowledge is power, and the power of AI, and I think our guest is going to agree, is something where we have only scratched the surface. And it’s probably limited as much as anything by hardware at this point as it is by human ingenuity and the ability to write code.
Mike Blake: [00:03:10] And so, my suspicion is that for a lot of us who are small business executives and owners, we may have written off or not paid attention to artificial intelligence because, you know, candidly and I’m guilty of this too, it sounds like something that only the big largest companies can afford. Right? AI is so expansive. And, we’re going to talk a little bit about the alphabet soup that goes into AI and how to make a little bit of sense from it. But it’s been around a long time now. It’s beyond, well beyond that early adopter phase or the cutting edge phase. Maybe it’s still in early adoption, but that means there’s plenty of room for AI to grow, to be creatively addressed, to be approached, and probably no two businesses are going to use AI exactly alike.
Mike Blake: [00:04:01] And, as usual, since I know almost nothing about the topic that we’re going to discuss, we’ve brought in an expert. And, joining us today is Charlie Wardell of Digital Cortex. Charlie is a technology entrepreneur, inventor, and consultant with over 20 years of experience in the field. He has a passion for innovation, which is showcased by patents related to big data and distributed computing, text analytics, and emotion detection in texts. He is also the owner of a provisional patent for a very unique FPGA that’s freely programmable gate array, for those of you scoring at home, hardware accelerator that brought the demand of financial institution back testing from 130 servers down to five. And, he also has a patent on a big data business approach.
Mike Blake: [00:04:46] Digital Cortex is the ultimate data processing and machine learning accelerator. They read anything, apply solutions, specific models and analysis, and put the results for you where you need them. With its combination of proprietary hardware and software, Digital Cortex delivers hyperscale data processing and inferencing performance. Multiple CPUs, FPGA, GPUs, and DPUs work together to enable you to achieve blazing fast speeds for your most demanding tasks that are focused on solving, once and for all, the scalability issues that keep meaningful insights hidden in large data sets. With Digital Cortex, you get line speed and hyperscalable access to those insights when you need them. Charlie Wardell, welcome to the program.
Charlie Wardell: [00:05:30] Thank you. Thank you for having me.
Mike Blake: [00:05:33] So, what is AI? Some people who think about artificial intelligence out there know a lot more about it and they actually know what it is. Others think back to the time they last watched a Terminator movie and they think artificial is used to go back and kill John Connor. I don’t think we’re there yet, but if we did, we wouldn’t know about it. How do you describe artificial intelligence to somebody who doesn’t have a Ph.D. in the field?
Charlie Wardell: [00:05:57] Yeah. So, you know, AI has been around for ages, right, since the ’60s. They’ve been trying to crack the AI code and make essentially have computers make decisions. Not to be confused with machine learning, which is a subset of AI that helps drive those decisions, but AI is essentially a technique by which decisions are being made whether a human is in the loop or not is irrelevant. And then, there are various forms of AI. You have symbolic AI. You have expert systems. You have neural networks and things like that that help you drive these decision-making processes. So it’s a complex topic with many facets. But what I hope to do on this call is boil it down to some of the practical as to what it means for small and mid-sized businesses.
Mike Blake: [00:06:51] So, in around artificial intelligence, you see or hear a lot the terms neural networks and machine learning. In fact, you just spoke of them, right? How do those three things interact with one another?
Charlie Wardell: [00:07:09] Okay. So, machine learning is the analysis of data in one of two forms. It is analyzing data where you’re either analyzing it in a supervised fashion, like there’s a human in the middle, right? We are providing data to a machine. That is what we call labeled. Here are examples of smiles or happiness, okay, and we provide as many different variations of that smile as possible, maybe in an image. Okay. So, that’s human-annotated labeled data. And then, the machine learns that these are smiles, these are frowns. That’s essentially one type of machine learning.
Charlie Wardell: [00:07:54] Another type is unsupervised. And you say, given all of this data, maybe cluster together the ones that look alike and do it on your own. And, that’s a clustering algorithm and that’s another form of machine learning. Both of which are used or can bubble up into an AI solution, but by themselves are not necessarily AI. You might think the fact that a machine can pick out a smile versus a frown is artificial intelligence. And, you know, I guess at a rudimentary level, it is. But it is not the AI that we’re talking about today where you have some smart drones being able to pick out the proper target, you know, in Ukraine, which is crazy AI. It’s pretty wild. So, that’s machine learning. AI is layers and layers of the machine learning that actually create a human-like decision. Right?
Mike Blake: [00:08:57] So, I might be completely off base, but I’ve often thought of artificial intelligence, like you said, going back, I would argue that artificial intelligence on some level has been around almost as long as computer programming has. Right? The second that they started letting you make if-then statements, that is a rudimentary form of artificial intelligence. Right? But where the machine learning comes in – and I love your smile analogy, so I’m going to take it, steal it and run with it – and that is that under a sort of a pure or plain vanilla AI framework. The programmer would have to tell in exacting detail the computer what a – what the characteristics of a smile or group of smiles or an epistemology of smiles looks like. Whereas under machine learning, you can show a bunch of facial expressions and over time it becomes good at understanding on its own what a smile looks like and it doesn’t have to be a separate algorithm that is fixed, that defines that smile rigidly. Is that a fair distinction?
Charlie Wardell: [00:10:05] Yeah. I guess, so really interesting. So, your analogy about the if-then statement is spot on. Back then, we called those expert systems. They were based on Prolog and lists some – I’m dating myself, but those expert systems were essentially if-then statements to the extreme, so many of them that it’s not humanly possible to code them all and maintain them all. And some of the best expert systems are used in the medical field where you interview a doctor and he may be a specialist in cardiology and you just interview him every single weekend over a cup of coffee until you pick his entire brain and you document these things as rules. Right? And then, you have a patient that comes to you and you type in his symptoms and it traverses all of this logic and all of these if-then statements and it says you have this. And the doctor looks and he thinks about it and goes, “Oh, it’s right. Holy smokes.” So, that’s a form of AI, right? That is an expert system AI.
Charlie Wardell: [00:11:07] Today, you have the smile analogy where the machine is actually picking up what a smile looks like. You’re not telling it any rules. It’s actually figuring it out and it’s like, “Wow, this – you told me these were smiles. So, I’m going to figure out why they’re smiles. Okay. Teeth are showing. Maybe, the mouth is wider or maybe the eyes are squintier, or maybe all of that stuff. I’m going to figure out why.” And that’s a different kind of AI.
Charlie Wardell: [00:11:34] What’s happening today and what should be happening today is the convergence of the two, right? Because together they’re better. And I can give you an example of a chatbot that I did. So, you have a chatbot. Let’s say it’s a mortgage application chatbot and people are saying, hey, I want a mortgage. And then, you have this thing traversing through the rules and parsing out that text and say, “Mortgage wants to know about a mortgage. Here’s my response.” It’s a canned response. And he says, “Well, do I need – how much is my down payment?” Looks up, answers. That appears to be AI, but that’s all this symbolic expert system-driven stuff. Then, they throw you a curveball and they say, “Hey, did you see the game last night?” Because they think they’re talking to a real person. That’s not in my decision tree. So, what do I do? I go to a neural network that was trained with the latest news. And I see game, scores and I’m able to pull that out and reply, right? So, now I’m doing the best of both worlds and I’m now making a real AI experience that is very different than the old school symbolic if-then statements. People are like, “Wow, how did it know that.”
Mike Blake: [00:12:53] You know, as I listen to you and even as I was doing research for this conversation, I think I’ve probably made a moron of myself. I mean, it’s more in an okay way, but I’ve probably been very polite and I’ve probably been very complimentary to basically robots that have given me customer service. Right? Because I try to – I do try to be empathetic with customer service. They have a tough job. They probably have people that call up and swear at them and threaten to blow up their houses and God knows what else. They’re not happy with the outcome. And so, I get good service. I try to be positive about it, just like I do in life. I try to be acknowledging of when good things happen. I’ve probably told at least one robot how much I think they did a great job and I love them. I think they’re just awesome, quote-unquote, people, right, if we’re honest about it.
Charlie Wardell: [00:13:46] Right.
Mike Blake: [00:13:46] Right.
Charlie Wardell: [00:13:47] Yeah.
Mike Blake: [00:13:48] Which shows us doing its job, right? Because it had, the chatbot in this case had such a human quality. The artificial intelligence was so well developed that indeed I had no conception that there wasn’t actually somebody busily typing on a window somewhere actually helping me.
Charlie Wardell: [00:14:06] Yeah. You know, and AI, it’s getting to the point where it is so unbelievable that you are getting to a point where you’re not really able to tell a difference. My entire resume – my entire resume, I wrote, and then I put it into this AI machine. There’s a few of them out there. And it rewrote it for me and it was amazing. I was like, “Yep. we’re going to clip that. They didn’t get that quite right.” And people would say, “Oh, my gosh, your resume is amazing.” And, it’s all factually true. Everything in there is factually true. But the embellishments that it made and the connected words that it used, it’s just absolutely mind-blowing. So, that’s just one aspect of AI that anybody can use in their business, this narrative generator. And it’s scary how awesome it is. It really is. It’s very awesome. It is.
Mike Blake: [00:15:07] So, let’s talk about the awesome because I’m not sure there’s a full appreciation of the awesome. I think a lot of the awesome is sort of hidden from view by design. In your mind, what are some of the most exciting recent developments in AI? What’s kind of new and neat that’s come out? And if you want to talk about the stuff you’re doing, that’s fine too. I’m familiar with it to some extent. Chris has briefed me. Or, other things too. But what’s really neat and new with AI right now?
Charlie Wardell: [00:15:34] Well, you know, let’s go with Ukraine right now, you know, which is, maybe people didn’t realize what AI could do from a military aspect. Right? So, you have these things called slaughter bots, right? They’re called killbots. And they’re this £6-drone that launches and it can travel like 6 miles and hover the air and it looks for targets. Now, you have a line, a caravan of, you know, heavy equipment, you know, enemy personnel. Well, out of all of those, which one should a dive bomb? Well, it’s going to look for the gas tanker, got to kill that supply chain. And it knows. It knows. I’m going to go for those first. Right? And, after I get rid of all of those, then I’m going to start getting these, and I’m going to do the missile battery next, and I’m going to do this next. That’s where AI gets – that’s where people can relate to say, because it’s in the news right now and say, “Oh, I get it. I understand what AI is doing now. I can discern and I can make decisions in flight, in real time, and do my job.”
Charlie Wardell: [00:16:00] From a business standpoint, on marketing – my wife has a business. It’s an e-commerce site. And, in that business, it’s made up of moms. Right? And, these moms have certain characteristics of the things they like, the things they don’t like, the things they buy, the things they don’t buy. You can upload your customer list to Facebook. And you can say, “Hey, Facebook, you have a billion people in your audience. What I want you to do is I want you to give me a new audience that is not my customer base but that looks exactly like my customer base,” from mathematical point of view, exactly, age, demographic, region, interests, and all this other stuff. And, now that becomes my target list for sending ads or messaging or email or whatnot. It’s called lookalike audiences, and it uses clustering technologies.
Charlie Wardell: [00:17:44] So, you have the one extreme where you can see that, wow, this is real AI. It’s autonomous and it is just doing its job. And those things cost, you know, $6,000 apiece as opposed to $6 million apiece. And then, you have lookalike audiences that help small and midsize businesses become a little bit more effective and who they’re targeting. Right?
Charlie Wardell: [00:18:05] Back in the day, you had to buy a list, got to buy a list. You had to tell them, “Hey, you know, give me you know, people in this age, this demographic.” You buy a list, you put a stamp on an envelope and you sent it out. Those days are gone. Right? And it’s so far more accurate that this is the day and age of AI.
Mike Blake: [00:18:26] You know, one of the – the Ukraine thing that you bring up, that’s for personal reasons, that’s a conflict I’m following very closely. And the AI that you describe brings up another very interesting point, which I’ve kind of wondered about, and that is that in that war, friend or foe detection has got to be extremely difficult because they’re basically using the same stuff.
Charlie Wardell: [00:18:54] Yeah.
Mike Blake: [00:18:54] Right? It all looks the same. It’s not supposed to be that way, right? Everything was built so that our stuff would look like our stuff. And their stuff looks like their stuff. But now there’s stuff and our stuff or the Ukrainian stuff all looks the same. Right? And I got to imagine there’s also an AI – there has to be an AI component to helping assist, to make sure there’s not a lot of friendly fire. And, it’s interesting that I’ve not heard of a single incident of friendly fire, of a significant incident of friendly fire yet in this war.
Charlie Wardell: [00:19:22] Yeah. And that’s where expert systems start coming in play, right, where you have a rule-based on top of it. Okay, I’ve done my job. I’ve analyzed visually. Here’s my target. Now a series of rules start happening, right? There was another project that we were working on where, you know, there are experts in theater that they’re in the military and they just know when something’s up. There’s a van parked on that corner. There’s a dead dog over on this corner. There’s a group of people over on this corner. And there’s an IED under the dog who’s whimpering or dead, and you go over there to help the dog, and boom. Right.
Charlie Wardell: [00:20:03] So, this scenario, right, this scenario, that’s all rule-based. You know, what they’re doing is they’re typing in all these rules. The intelligence gathering is trying to type in environmental rules and then the expert system type AI will take over in cases like that. Others are visual. Others are audio. Others are streaming data where it’s such high velocity that you’re kind of stuck in having the machine make the decision for you in real time. And, that’s where things like the Digital Cortex comes in because the amount of data is so enormous that you’ve got a hyperscale and hyperspeed the processing of this data, and you can’t do it in the cloud, right? I cannot have this thing. It’s got to be in my backpack. It’s got to be on this machine. Can’t do that from the cloud.
Mike Blake: [00:20:53] So, what are the most common applications of AI right now? Is it all big data analytics or are there other applications that maybe are more visible that our audience would be familiar with?
Charlie Wardell: [00:21:10] Well, you’re going to see more and more of this writing style, help-me-write books and blog posts, and automatically you just seed your thoughts in it and it’ll ghostwrite an entire book for you. You’re going to see. That’s happening now. You can Google it. I’m not touting any one technology over another, but you can go find them and trial for 30 days. They are unbelievable.
Mike Blake: [00:21:37] I’ve seen the ads for that. Do they actually work?
Charlie Wardell: [00:21:39] They work.
Mike Blake: [00:21:40] They work.
Charlie Wardell: [00:21:41] They work. They are incredible. Then, you know, other aspects of AI, you know, obviously, in a practical sense, it’s – think of a camera hanging out in a WalMart parking lot and a guy taking out a gun out of the back of his truck. Is he returning it, or is he going to open fire on somebody? Is this an actual threat or is this just a customer that’s returning his gun, right?
Charlie Wardell: [00:22:14] And, given enough scenarios, right, given enough scenarios where we can actually train AI and all of the, what we call labeled data, it can make guesses and the guesses return percentage of probability. And that percentage of probability, once it crosses a threshold, then requires action to be taken. So, you’re going to see it in all aspects of life. And I know people are afraid of it, but there are good there are good aspects of AI that can help humanity, obviously.
Mike Blake: [00:22:49] Yeah. No, I think you’re right. I mean, you know – the thing about AI is that it never gets distracted, never gets bored, never gets arrogant and thinks it knows everything, right? And so, for things like things that require checklists, whether it’s prepping for surgery or landing an A350, AI’s not doing that. Yeah. Although I think AI probably could land a plane. We just never got on a plane that didn’t have a pilot in it.
Charlie Wardell: [00:23:22] Well, there’s AI – there’s AI Assist. Yeah, there’s AI Assist. And, this is where it’s human in the middle. Right? Trust your instruments. Trust your instruments. How many flights have gone down because they didn’t trust their instruments?
Mike Blake: [00:23:37] Oh, yeah. Yeah. Literally, pilots are fighting planes into the ground.
Charlie Wardell: [00:23:42] Yeah, exactly. Now, with AI – AI – see, AI is getting data that you can’t see, comprehend or process because it’s looking further down the road. It sees that there’s – it knows there’s turbulence ahead. Why? Because someone else reported it. It knows the wind speed. It knows, so it’s figuring stuff out, right? So, it’s going to have to take a lot of surrender to surrender to these machines and to totally trust it. And, machines have failed us miserably in the past. So, it’s going to be a while, but it’s definitely.
Mike Blake: [00:24:21] So, this is an impossibly broad question, but I have to ask because we have to start somewhere. Somebody is listening to this podcast or will be listening in when it gets published and they’re saying, “Okay. Hey, I can do all these things. I’m probably not knowingly using it a great deal in my company.” How do you get started? Where do you go from there, from saying, I’m kind of interested in getting AI into my company to have it actually do something useful for it?
Charlie Wardell: [00:24:52] Yeah. So, every company has their different aspects of AI, right? If you’re marketing product and services and things like that, and you’re an e-commerce site, there’s just tons of AI available to you in the form of lookalike audiences and market basket analysis to figure out if you buy this and most people buy this along with it and make recommendations. And Amazon’s been famous for that. You know, if you’re a bank, maybe you’re using AI to do some risk mitigation, you know, maybe you have all the people that defaulted and all their properties at default and you’re looking at this person’s characteristics and you have a default probability.
Charlie Wardell: [00:25:39] You know, most of it is related to the data that you’re collecting. A lot of it is is about lookalike audience. It’s about churn probability. These customers have the, hey, I know historically that a customer that visits my support site three times in a single month has called up and asked specifically about his contract price and has basically stop doing X, Y, or Z is likely to churn, right?
Charlie Wardell: [00:26:15] So, those are the types of things that businesses are doing now. Now, what’s typically required in order to get to that level of analysis is that you have a data scientist who has a hypothesis or you have a mandate from a company that says, “Hey, I want to identify my high churn risk customers.” Then, you get a data scientist to say, “Okay, give me a list of all the customers that churn and let me find out what’s in common with them,” and then runs it through these steps of trying to identify the actual machine models that would predict it with great precision and great recall. So, it usually starts there.
Mike Blake: [00:26:53] So, that suggests to me, correct me if I’m wrong, but that suggests to me that a prerequisite step for adopting an AI centric or AI adjacent strategy is you’ve got to have good data collection in place.
Charlie Wardell: [00:27:08] Absolutely.
Mike Blake: [00:27:10] Right? If you don’t have the data asking any – computers are no better at making decisions based on no data than we are.
Charlie Wardell: [00:27:17] That’s right. And, you remember the phrase, GIGO, right, garbage in, garbage out.
Mike Blake: [00:27:21] Sure.
Charlie Wardell: [00:27:22] Yeah. So, you know, it’s – we’re – I’m on a project right now where we have all of these customers calling in and these are accounts. And, I’m able to cluster the accounts together and say, these accounts look like this and this account looks like this. But what we’re trying to do is we’re trying to find out, okay, they’re service calls that they’re calling in about each product or platform that they’re calling in about. What is the tie between their overall happiness and the calling in that they’re doing on these products and actually seeing if there is upsell potential into new products? Is there expansion opportunity? Is there – are they about to churn or are they – so, the more data that I can feed this machine about that customer and about their interactions across my organization, the better. Now, the challenge is in these organizations. All this data is disparate. They’re in silos and they don’t connect. There’s no one single ID that connects this and this and this and this. They’re legacy systems. And that’s typically what the big challenge is.
Charlie Wardell: [00:28:27] And then, the next big challenge is, I have all this data and I can’t process it fast enough to make any difference because this is a wash,rinse, repeat cycle over and over and over again until you get to the model that does the prediction accurately. So, it’s an expensive proposition in some cases. But these off the shelf things, like lookalike audiences, that most of these social platforms and ad platforms have, they’re set it and forget it. You upload your list. It handles everything for you. So, you don’t have to really get involved in doing anything.
Mike Blake: [00:29:02] So, that leads me to a couple of questions. I hope I remember to ask them both, because I think they’re both important. The first question is, it seems to me, based on what you’re saying, that in some cases a move to heavy reliance on AI, whatever that may be may also require an accompanying culture change. Right? Because if you’re not used to collecting data, if you’re not used to, you don’t have a culture that’s willing to share data, you have little fiefdoms, you may even have a culture that resists accountability. And we know there are cultures out there that do that. And data is kryptonite for lack of accountability. There may be a culture change that needs to accompany this [inauidible] to work, right?
Charlie Wardell: [00:29:53] Well, so I think back to the example where I think it was Microsoft that put out this amazing chatbot. And the internet went crazy teaching the chatbot how to become a fascist. Right? The chatbot actually became rogue. They had to take them down. So, yeah, there’s a big cultural aspect of AI as well, because –
Mike Blake: [00:30:18] A fascist chatbot. I hadn’t heard of that. I can’t wait to Google that after this interview and hopefully Homeland Security will not be paying me a visit, but –
Charlie Wardell: [00:30:27] It’s crazy, you know, because the Internet is a thing of its own, right? And, AI learns what you teach it. And if you teach it, if enough people get together and start telling it truths that are not necessarily truths, it’s wrong.
Mike Blake: [00:30:47] Sure. And, was that an act of sabotage from within Microsoft?
Charlie Wardell: [00:30:52] No. It’s just the Internet having fun. Internet trolls having fun with it.
Mike Blake: [00:30:56] Okay. But what about within a company again? It seems to me that the move to AI, if you’re not already a data-centric company, if you’re already a company, that’s not – that struggles with internal transparency, sharing and teamwork, AI probably is not going to work all that well for such a company unless you kind of address those underlying cultural features.
Charlie Wardell: [00:31:25] That’s true. So, most of the data that’s curated is internal and well guarded, and they understand that there needs to be a big effort in protecting your biggest and most important asset, which is your data. Right? And, it’s only up until recently that people understand that their data is everything. Every company that I’ve been talking to, every single one, no matter how large or small, they want to be a data-driven business. Right? And, getting access to that data and treating it like gold is really, really important. And, they’re starting to get that part. People are just starting to embark on their AI efforts now because they’re only starting to grapple with the fact that we have to make an investment in curating our data in a way that is clean and trustworthy and accessible.
Mike Blake: [00:32:19] And so, I want to go back and ask the other question about that, which is, is AI in some fashion, is that in the realm of affordability for a small business? Are there models, other pieces of AI where a small business is doing, let’s say 1 to $10 million of revenue a year could reasonably take advantage of this technology? [Inaudible]
Charlie Wardell: [00:32:45] Yeah. Yeah. AI is white hot right now. The market for students coming out of the university, wanting to be developing machine learning algorithms and AI and things like that. They’re available, you know, for a reasonable salary. You can get reasonable AI work that will definitely help you drive good decisions in your business. And then, there are applications that you can download for $30 a month and have it write your your daily blog. You know, seriously, it’s that crazy.
Charlie Wardell: [00:33:19] And then, things like look alike audiences, if you’re doing ad spend and stuff like that that’s free. They just want your ad spend, right? So, for a once – for a milllion dollar company to get into the game, it’s not hard. And, those things will make you a $2 million company and the $3 million and then eventually you’ll have a team of data scientists doing amazing things. But, yeah, the barrier to entry has definitely reduced, over the last few years has definitely reduced.
Mike Blake: [00:33:52] Now, you’ve touched on this a little bit, and I want to make this explicit because I do think it’s important. If you’re going to undertake AI in a serious way, do you need to think about having a captive AI specialist or big data specialist on your team? And is that even possible? I mean, those people are very hard to hire anyway, even if you wanted to. But is that a prerequisite for success using AI tools?
Charlie Wardell: [00:34:20] It depends on the AI. Yeah. Yeah. So, I mean, if I’m, you know, just wanting something to write my blogs and my responses and my creative, no, right? There are applications out there that do that. But if I have a hypothesis and I have all this data and you definitely do need some sort of architect, some sort of data scientist that knows how to get there from here. There is a part of machine learning that is a black hole that we all fear. It’s called feature engineering. And, you have all of these attributes of data and only a handful of them make a difference. Right?
Charlie Wardell: [00:35:02] I’ll give you an example of – so, I’m big in text analytics and I would analyze text and try to pull out all the topics out of text and I curated a list of texts that were very pro a product, very pro, this product. And, I identified the language that made it pro the product. Now, think about the iPhone when it first came out. “Oh, my gosh, this is amazing. This is a game changer. You know, I’ve never seen anything like it.” or the iPad. “Hey, now I don’t have to carry my laptop with me wherever I go.”
Charlie Wardell: [00:35:40] So, there is a language of this wow. Right? There’s this language. So, I’m able to tease out this language and identify all of the features so that when a new tweak comes in, I can compare it to that model and say, is that wow factor in there or not? No. But here’s the interesting thing. Out of all the features that I found, and I added them all in, the sentiment of the text, the length of the words, the number of periods, commas, exclamation points, the number of curse words, the date, the length of the author’s email, there was one feature that made it really interesting, and that was the number of sentences, was an indicator to how prolific this product experience was for this person. It was the number one feature in machine learning, and nobody would have ever thought that unless the machine figured it out. The machine figured it out. It wouldn’t be something. I just threw it in as a happenstance. Right? Number of sentences.
Mike Blake: [00:36:51] Where in your mind is AI not being utilized to its fullest potential? Where you see as a sector or an application, you say, “You know what? I’m surprised more people are doing this.”
Charlie Wardell: [00:37:03] You know, schools, how we teach our children, we don’t all understand. Right? I think the schools should be looking at clusters of students and figuring out how best to hone curriculum for those types of students. We learn differently. I think that – you know, everything from your spending patterns and how you optimize your budget and where you should be investing, I think those types of things are very ripe for consumer programs where you feed in the characteristics of your family, your spending, your goals, and it comes out with a plan and says, follow this plan and you’ll get to where you’re going. And, I think there’s a lot of consumer activity that can happen in these just turnkey applications.
Mike Blake: [00:38:01] So, how do you evaluate AI platforms? Let’s take the lookalike audience platform. You brought that up a number of times. I presume that’s important and fairly widespread and I’m assuming there’s more than one source you can go to. How do you evaluate among competing or I guess what will be presented to the market as comparable platforms? How do you evaluate that? Is there a checklist? Are there certain things that sort of top three or top five things that you need to be looking at? You need to hire an external specialist or consultant that really understand this stuff. How do you go about doing that?
Charlie Wardell: [00:38:52] Lookalike audience. You know, they’ve really dumbed it down so that anybody can use it. But the success of the lookalike audience really determines – it’s really how much of the features do have you collected so that it can match up against. So if the only thing I have is gender and age, and I say give me a lookalike audience for gender and age, it’s a coin toss as to whether or not I’m going to hit the right demographic. But if I have gender, age, the car you drive, you know, the number of friends in your social sphere, the part of the world you’re in, the hobbies you do, and all of this other stuff, I’m going to radically change my marketing return on investment. Right?
Charlie Wardell: [00:39:44] So, what they’ve done is, they made it so easy. You upload a CSV spreadsheet to our platform and we’re going to carve out your lookalike audience, but give us as many of the features as you possibly have, because we have them all. They have them all and more. Right? You’d be surprised as to what they have. Right? So, what you’re doing is you’re uploading what you have and they’re matching it with what they have and are carving it out. So, very simple, very easy. And, most platforms to this day, specifically Facebook, all have this type of lookalike audience.
Mike Blake: [00:40:13] So, as we all know, looking back on the last two years, the world has just changed dramatically. Our relationship, among other things, with technology has changed dramatically because we had to. We had this sort of shock therapy in terms of digital transformation. Now, that we’re in this what I call a trans-pandemic period, I don’t think we’re out of it, but we’re not, and I’m not sure where we are so I’m calling it trans. Looking back, where did AI contribute to making that less terrible than it otherwise would have been? And then, if I can also ask this, I know this is a complicated question, but you can handle it, and that is, what opportunities for AI have been revealed or exposed by the COVID experience in your view?
Charlie Wardell: [00:41:15] Wow. Well, it may go hand-in-hand. I’ll answer your second question first. But we all know, and we spent so much time listening to what fake news was, right? And, you know, curating data and actual correct data is paramount to having good AI. So, I think that when you have such a divisive country in what they’re sharing in this sentiment and it becomes very nebulous and this is where AI failed you. This is like what is it about, you know?
Charlie Wardell: [00:42:11] But, you know, where AI succeeds is looking at the cellular level of maybe this disease state and looking at the characteristics and matching it up with others to to say there’s a similarity between these two and we’ve already figured out how to solve this one and it’s very similar and how we can apply some similar therapies to this and try it out and see if it works. That’s where it really could help us. So, on one hand, in the pandemic, you could see how it hurt. On the other hand, you could see very clearly how it helped. So, I think I got both your questions. Did I miss one?
Mike Blake: [00:42:59] No, no. I think you did. You answered it in a way I did not expect, but that didn’t make it bad. I think it’s a very – that’s a very thought-provoking answer, because in my view, I’ve got to be careful because I don’t want to be partisan the way that I express this. In one fashion or another, we have been flooded and continue to be flooded with – call it- anti-data. Right? Now, we’re in a a society now where gaslighting is a contact sport now and just like your analogy or your example of Microsoft chatbot being trained to be a fascist basically because of a big cyber prank, right?
Mike Blake: [00:44:01] Yeah. I do think that the drawback of AI, and this isn’t unique to AI, it’s really technology in general. Right? Technology is an amplifier first and foremost. Technology is basically a lever when you really boil down to it, or a power tool. So, something that’s good and productive be amplified tremendously by technology, and something that is destructive can also be and is amplified by technology. Right?
Charlie Wardell: [00:44:38] And, whether you’re a bot or whether you’re a person, you cannot possibly make – I shouldn’t say you can’t possibly – you can’t reliably and sustainably make good decisions. You can lock into a good decision even with bad data. That does happen. But you can’t be a sustainable and reliable decision-maker if the data on the front end is bad. But now what happens, I’ve posted about this before, particularly the way that the news and the social media business models are, it’s no longer about informing people. It’s about getting people riled up because riled up people tend to be better customers. They tend to watch through your commercials. Right? And they tend to spend more. They tend to pay more. They’re a much more valuable audience.
Charlie Wardell: [00:45:31] You’re absolutely right. You could see this in technologies like TikTok, where it’s bringing things up to you that are somewhat controversial and it may not be what you’re interested in, but it gets a lot of the stickiness. And then, when you start looking at all of the reactions, you start seeing that you’re in a bubble. If this is your only platform, you’re in a bubble. You think the world is exactly like what was just presented to you. And it is not. It is really not.
Charlie Wardell: [00:46:04] So, there’s got to be a gatekeeper of truth in AI. There’s got to be. And you call them fact checkers now, right? There’s got to be a move – with AI, the responsibility is truth. There’s got to be truth. And I don’t think we’re there. I think we’re far from there.
Charlie Wardell: [00:46:25] Now, into your internal organization, you can guarantee the truth, right? You could say this is the facts. These are customers that left me. These are customers who love me. This is where we screwed it up. This is where you have facts, you have truth. And then, you could trust that AI. But when you start coming into this social sphere, it’s going to represent what humanity looks like today. It’s just going to become whatever it’s being fed.
Mike Blake: [00:46:53] Well, I mean, definitionally, it’s a feedback loop, right? That’s what it’s designed to do. And, maybe that’s a flaw. Not a flaw, but that’s just a – it’s a point where we need to just be aware. And, we’re getting a fascinating social discussion here. Right? But perhaps an area of evolution for AI, and maybe this is already happening. And you tell me this, we’ve already got this. But one area of AI that has to, I think has to evolve is there has to be some sort of emergency brake that just sort of cuts off the feedback loop or it doesn’t go off an artificially intellectual deep end and go into a feedback loop that just sort of drives the AI off the rails and becomes and perpetuates more extreme decision-making.
Charlie Wardell: [00:47:46] You’re absolutely right. And, this is probably one of the scariest factors of AI in use is what happens because there are some malicious people out there. They’re just trolls and they don’t understand the impact of what they’re doing. Now, from a social perspective, I don’t think it’s going to make a difference as to whether an AI assists a doctor in atrial fibrillation ablation. It’s not going to make a big difference because completely different kind of AI. But from a social perspective, yeah, it’s a whole new can of worms that we haven’t even begun to navigate through yet.
Mike Blake: [00:48:34] So, let’s bring it back to business for a second even though I could talk about this for three hours, and maybe you could too but our listeners don’t want to listen to it for three hours. What are the risks of of bringing AI into a business? What could be unintended consequences? What could go wrong?
Charlie Wardell: [00:48:51] All right. So, I’ve been doing data warehousing for many years, close to 30 years. And, there are some key indicators as to why data warehouses fail. Lack of executive sponsorship, not understanding the technology or choosing the wrong technology, not understanding what you’re getting into and the commitment required to get into it. Lack of adoption, dirty data. These types of things all apply to AI initiatives today. Thirty years later, they still apply. Seventy percent of data warehouses failed because of the things I just mentioned.
Charlie Wardell: [00:49:33] Well, if you’re going to embark in an AI initiative, you have to have executive sponsors that say we are going to be a data-driven organization. Right? And if they say that, that means we are going to make an effort to make sure our data is trustworthy and properly cleansed and integrated. And, we’re going to have one source of the truth so that when we do develop our AI models, that we can trust our AI models and we are going to reasonably expect realistic expectations of AI. Is it 86% where we make a decision or does it have to be 95% in order for us to trust our AI models?
Charlie Wardell: [00:50:18] And it is a continuous, nonstop endeavor of constantly moving forward. So once you start, you’re always continuing to better it, right? So, if you’re taking it from a perspective of this is how I am going to be transformational in my business, it comes with a certain understanding that you have a – this is a marathon, it’s not a sprint. You want to sprint, go download that app to write your blog. You’re an AI. You want to be transformational, you have to be willing to run the marathon.
Mike Blake: [00:50:55] I’m talking with Charlie Wardell. The topic is, should I use artificial intelligence in my business? I want to be respectful of your time, so I only have time for a couple more questions. But one thing I want to get out of you, because I think your answer is just going to be awesome, that is, what’s coming ahead? What are some future applications of AI that you see that aren’t in use yet but we may see as viable in the next 5 to 10 years?
Charlie Wardell: [00:51:28] I think the obvious one is driverless cars. Logistics and supply chain, you know. I don’t understand the levers that are moving our supply chain problems right now. I just don’t understand. It makes no rhyme or reason to me that we have this supply chain problem.
Charlie Wardell: [00:51:52] Because we’re given a different reason. Every time something goes bad, there’s a different reason.
Charlie Wardell: [00:51:56] That’s right. But being able to predict manufacturing and supply chain and things like that, to be fully optimized in the supply chain, I think that’s another aspect that we’re going to see a lot of AI. Obviously, fintech. And, fintech has its problems, right? You have to be able to explain your AI. And, AI does not necessarily lend itself to explainability all the time. You got this black box of this machine doing something and figuring it out and comes out with an answer. And you don’t know how it came out with that answer. But it did and it’s right. I think there’s going to be some changes that you’re going to start seeing more AI used in the financial markets that is more widely accepted.
Mike Blake: [00:52:51] That’s a really interesting observation. So, I’m the world’s lousiest accountant, which is even though I work for an accounting firm, I don’t do any accounting. And, they’re smart not to let me do that. But that brings up a very interesting point, which I’ll bet you some smart accountants are thinking of and probably some of our people at Brady Ware are thinking of, which is, how do you audit data that is AI generated? Right? There’s a recognition in the accounting literature and the literature of what I do in business valuation and informed professional judgment is a recognized piece of the overall analytical story. But what if the informed professional judgment is my tablet or it’s in the cloud or it’s an app? How do we reconcile ourselves to that? I don’t expect you to have an answer for that, so it’s a rhetorical question generally, but it gets to the heart, I think, of that next level is, how do you make judgment? How do you make artificial judgment transparent?
Charlie Wardell: [00:54:01] Yeah. Well, I’m not sure that I’ve seen that aspect of it right now. I think people are more trying to figure out what the answers are, and we’ll deal with that a little bit later. But think about for a moment like all the CEOs that are doing earnings calls at the end of the year or every quarter, and you have 20 years worth of earnings calls from a CEO or an executive. And I train my model as to the cadence of his narrative. And then, I see a deviation, or the machine sees a deviation into what he’s saying is forward looking statements, so to speak. And, I start suspecting there may be deception. And maybe the first time, I was right, and maybe the second time is called reinforcement learning. The more the machine is right, the more right it becomes. Right? So, there are aspects of that that are pretty interesting right now, and that is auditing. Right? Auditing records of what people are saying. How do you transparently audit? I’m not 100% sure. How do you know that the data that is generated is artificial, if it’s speaking the truth?
Mike Blake: [00:55:36] Well, whether the data is generated is artificial, I think is beside the point. It’s really just understanding. You know, it’s either – it’s a combination of understanding how the AI reacts to and interprets that data. And then, asking the bigger philosophical question, again, this gets into the three-hour seminar on the quad kind of thing, but it gets into the question of, what an AI or does AI have the capacity to synthesize and interpret that data the same way that a human being would if it had the computing capacity to actually process it? And is that even the appropriate standard? At what point do we just say, you know what, not only can a computer process more data more quickly and more comprehensively without error, but also the computer just has better judgment. Right? And, that question – I’m sure that question’s been positive. Somebody has written a dissertation on that at some point. But it’s going to move out of a dusty old dissertation in someplace and some of these three-and-a-half inch floppy disks and into a really important practical question that has to be solved, or otherwise AI is just going to be permanently handcuffed.
Charlie Wardell: [00:57:02] Yeah. And it’s going to go back to the quality of the data and is the data non-biased? Is the data trustworthy? And it – here’s the thing about AI, you know, as a human, you can run through a few scenarios. Right? And AI can run through a few hundred models simultaneously. It’s like the hurricane models, right? You see the hurricane models and they all converged. And then, you have confidence that, yep, it’s going to hit Tampa. Right? They all converge. And it’s not just one model. So, what’s going to happen is you’re going to have many, many models and they’re all going to converge and they’re all going to say, yep, morning, you know, this is what we think. And, sooner or later, like, we – sometimes we’re just shocked at how the weather is predicted. And other times we’re just like, what were they thinking? Right?
Mike Blake: [00:58:00] Right.
Charlie Wardell: [00:58:01] It’s all about the data, right? It’s all about the data. So, it’s a little – I think a little easier than predicting the weather. When you have 100 models and you have your data and you can run it through all these scenarios simultaneously and they all come up with the same answer, you need to listen.
Mike Blake: [00:58:19] Charlie, this has been a great conversation. We didn’t even get to all the questions and I anticipated that would be the case. That’s okay. But there are questions I’m sure that people, our listeners, would have wished that we had discussed or would have or wished that we would have spent more time on. If somebody wants to follow up with you about discussing using AI in their business, how to formulate a business strategy around it, can they contact you for more information? And if so, what’s the best way to do that?
Charlie Wardell: [00:58:47] Yeah. They can reach out to me on email. I’m charlie@digital-cortex.io, or my partner in crime, chris@digital-cortex.io. And, yeah, we love talking about this stuff. I didn’t get to speak about the Digital Cortex product and its revolutionary aspects of how it’s going to change the game. But that’s yet to come. We’ll have another podcast specifically on that one because that’s exciting. That’s what I’m – that’s my passion project.
Mike Blake: [00:59:20] Sounds good. Well, I think people will be visiting your website once they listen to this conversation to learn more at any rate. So, that’s going to wrap it up for today’s program. And I’d like to thank Charlie Wardell so much for sharing his expertise with us.
Mike Blake: [00:59:34] We’ll be exploring a new topic each week, so please tune in so that when you’re faced with your next business decision, you have clear vision when making it. If you enjoy these podcasts, please consider leaving a review with your favorite podcast aggregator. It helps people find us that we can help them.
Mike Blake: [00:59:51] If you would like to engage with me on social media with my Chart of the Day and other content, I’m on LinkedIn as myself and @unblakeable on Facebook, Twitter, Clubhouse, and Instagram. Also, check out my new LinkedIn group called Unblakeable’s Group That Doesn’t Suck. Once again, this is Mike Blake. Our sponsor is Brady Ware & Company. And this has been the Decision Vision podcast.